Organizers:
Mitchel J. Colebank (University of South Carolina), Vijay Rajagopal, The University of Melbourne, Australia
Description:
Cardiovascular models are now recognized as a potential frontier in the development of personalized models and digital twins. This new excitement in the field is fueling new strides in mathematical and computational approaches to describe cardiovascular function across different temporal and/or spatial scales. In addition, new multisystem models accounting for how the heart and vasculature interact with other organ systems are being developed in combination with tools located at the heart of data science. Thus, this minisymposium will focus on the development of cardiovascular models that mimic cardiovascular function across spatial or temporal scale, new models that couple the cardiovascular system with other physiological systems, and new innovations in data-driven solutions to modeling cardiovascular phenomena. Potential topics include: - Multiscale models of cardiac and vascular function; - Computational approaches to cell-tissue-organ level function; - Mathematical coupling of cardiac and vascular mechanics; - Modeling autonomic control and neurovascular function; - Simulating tissue growth and remodeling; - Multisystems models of cardiovascular-organ interactions; and - Physics-informed data science approaches to cardiovascular science
Mette Olufsen
North Carolina State University"An uncertainty aware framework for generating vascular networks from imaging"
Sara Johnson
University of Puget Sound"Modeling Microglial Response to MCAO-Induced Ischemic Stroke"
Simon Walker-Samuel
University College London"Using physics-informed deep generative learning to model blood flow in the retina"
Mitchel Colebank
University of South Carolina"Effects of vasomotor tone on systemic vascular wave reflections"
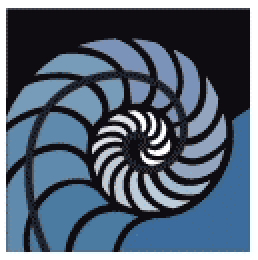