Organizers:
Keisha Cook, Anna Nelson (Clemson University), Alessandra Bonfanti (Politecnico di Milano) Giulia Celora (University of Oxford) Kelsey Gasior (University of Notre Dame) Qixuan Wang (University of California, Riverside)
Description:
In many cell and developmental processes, both modeling and data analytic approaches are necessary in order to generate useful modeling predictions to guide the design of further experiments for both validating and improving biological insight. There is an increased understanding that the application of machine learning methods can also be used to enhance common data-driven modeling techniques, including parameter and equation inference, classification, and sensitivity analysis. The speakers in this session will discuss how differential equation models, stochastic models, and methods from machine learning can be combined to address questions related to cell growth, intracellular transport, cell differentiation, cell migration, and tissue development. The speakers will highlight current research progress and challenges associated with combining modeling and inference approaches in cell and developmental biology.
Khanh Dao Duc
University of British Columbia"Optimal Transport based metrics and statistics for quantifying cell shape heterogeneity"
Peijie Zhou
Peking University"Towards AI Virtual Cell Through Dynamical Generative Modeling of Single-cell Omics Data"
Amanda Alexander
University of Houston"Persistence of plasmid DNA in spatially organized bacterial populations"
Grace McLaughlin
University of North Carolina, Chapel Hill"Modeling Asynchronous Nuclear Division in Fungal Cells"
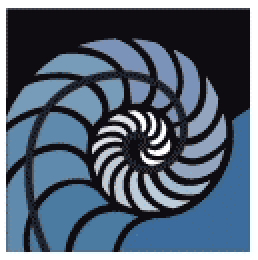