Share this
Holly Huber
University of Southern California"Multiscale Probabilistic Modeling - A Bayesian Approach to Augment Mechanistic Models of Cell Signaling with Machine-Learning Predictions of Binding Affinity"
Chongming Li
Queen's University Department of Mathematics and Statistics"Well-Posedness and Stability Analysis of a PDE-ODE Model for the Evolution of Bacterial Persisters"
neda khodabakhsh joniani Mrs
She"A Voronoi Cell-Based model for Corneal epithelial cells"
Shikun Nie
UBC"Estimating Rate Parameters in Super-Resolution Imaging via Hidden Continuous Markov Chains with Discretized Emissions"
somdata sina
INDIAN INSTITUTE OF SCIENCE EDUCATION RESEARCH (IISER) KOLKATA, INDIA"using networks for modelling three-dimensional structures of proteins"
Nathan Smyers
University of North Carolina at Chapel Hill"From Data to Dynamics: Uncovering Cell Signaling Networks with Physics-Informed Machine Learning"
Anna Nelson
University of New Mexico"Modeling mechanisms of microtubule dynamics and polarity in neurons"
Dietmar Oelz
The University of Queensland"Mechanochemical pattern formation in Hydra"
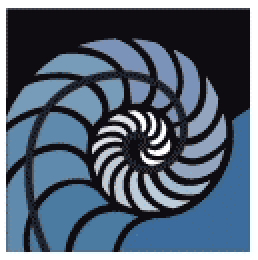