Share this
Silvia Berra
IRCCS Ospedale Policlinico San Martino, Genova, Italy"In-silico modeling of simple enzyme kinetics: from Michaelis-Menten to microscopic rate constants"
Ismaila Muhammed
Khalifa University"Data-driven Construction of Reduced Size Models Using Computational Singular Perturbation Method."
John Vastola
Harvard University"Bayesian inference of chemical reaction network parameters given reaction degeneracy: an approximate analytic solution"
Adelle Coster
School of Mathematics & Statistics, UNSW, Sydney Australia"Cellular protein transport: Queuing models and parameter estimation in stochastic systems"
Clark Kendrick Go
Collaborative Analytics Group, Department of Mathematics, Ateneo de Manila University"Exploring Mathematical Techniques in Collective Behaviour and Decision Making in Animal Groups"
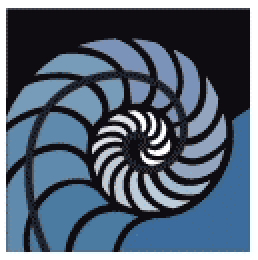