Share this
Siti Maghfirotul Ulyah
Khalifa University, Abu Dhabi, United Arab Emirates"Estimating the Growth Rate of Tumor Cells from Biopsy Samples Using an Extended Mean Field Approximation"
Hooman Salavati
Ghent University"Patient-Specific MRI-Integrated Computational Modeling of Tumor Fluid Dynamics and Drug Transport"
Rachel Sousa
University of California, Irvine"Identifying Critical Immunological Features of Tumor Control and Escape Using Mathematical Modeling"
Simon Syga
TUD Dresden University of Technology"Evolution of phenotypic plasticity leads to tumor heterogeneity with implications for therapy"
Alexis Farman
UCL (University College London)"Enhancing immunotherapies: Insights from the mathematical modelling of a microfluidic device"
Magnus Haughey
Barts Cancer Institute"Extrachromosomal DNA driven oncogene spatial heterogeneity and evolution in glioblastoma"
Luke Heirene
University of Oxford"Data Driven Mathematical Modelling Highlights the Impact of Bivalency on the Optimum Affinity for Monoclonal Antibody Therapies"
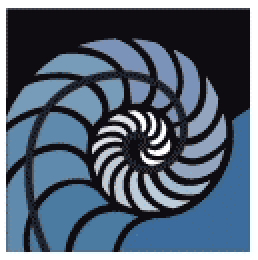