Share this
Ashlee Ford Versypt
University at Buffalo, The State University of New York"A Multi-Cellular Network Model Predicts Changes in Glomerular Endothelial Structure in Diabetic Kidney Disease"
Mojgan Ezadian
Lindi Wahl, Western University"A Continuous-Time Stochastic Model for Mutation Effects in Microbial Population"
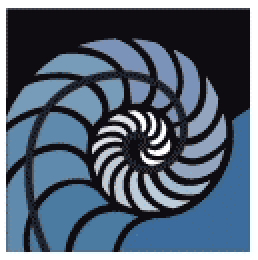