Organizers:
Abba Gumel (University of Maryland), Alex Safsten, Alice Oveson (both University of Maryland)
Description:
The recent COVID-19 pandemic has highlighted the critical role human behavior plays in the dynamics and control of infectious diseases. The behavior changes with respect to the adherence or lack thereof to public health intervention and mitigation measures during this pandemic were triggered by factors such as the unprecedented burden of the disease, the epidemic of disease-related mis(dis)information, fear, polarization, peer influence, poor quality and inconsistency in public health messaging, etc. Specifically, epidemiological models that do not explicitly account for these behavioral changes were seen to generally fail to capture the correct trajectory and burden of the pandemic (thereby not being able to make realistic or accurate forecasts). This minisymposium brings together an interdisciplinary team of researchers to discuss and share ideas on the recent advances in designing, validating, and analyzing mathematical models that explicitly incorporate human behavior and socio-economic factors, and use these models to contribute to public health policy for controlling and mitigating the spread and burden of the disease. Some of the topics to be addressed include metrics of human behavior changes, the role of heterogeneity in compliance to public health intervention and mitigation measures, the influence of social networks, the impact of mis(dis)information, and risk perception.
Mallory Harris
University of Maryland"Risk (Mis)estimation and Population Heterogeneity Shape Infectious Disease Dynamics"
Christian Parkinson
Michigan State University"Optimal Control of a Reaction-Diffusion Epidemic Model with Noncompliance"
Zitao He
University of Waterloo"From Sentiment to Spread: Homophily and Early Warnings in Epidemic Dynamics"
Alice Oveson
University of Maryland"Modeling Racial and Age-Structured Transmission Dynamics with Empirical Contact Data"
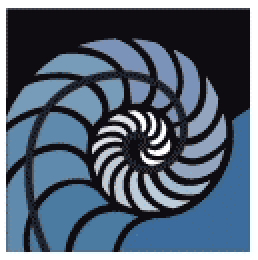