Share this
James Holehouse
The Santa Fe Institute"The Origins of Transient Bimodality"
Anthony Pasion
Queen's University"Long-Lasting and Slowly Varying Transient Dynamics in Discrete-Time Systems"
Elmar Bucher
Indiana University / Intelligent Systems Engineering"PhysiGym : bridging the gap between the Gymnasium reinforcement learning application interface and the PhysiCell agent-based modeling framework"
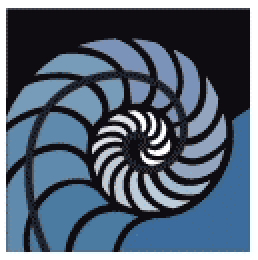