MS03 - MFBM-02
Bayesian Applications in Mathematical Biology
Tuesday, July 15 at 10:20am
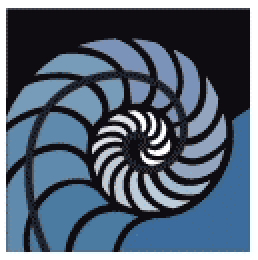
Organizers:
Daniel Glazar (Moffitt Cancer Center & Research Institute), Renee Brady-Nicholls, Moffitt Cancer Center & Research Institute
Description:
Development and employment of models in mathematical biology often involve tasks such as parameter estimation, uncertainty quantification, identifiability analysis, sensitivity analysis, and quantification of intra- and inter-subject heterogeneity. Bayesian inference methods naturally lend themselves to fulfill each of these tasks by defining distributions which characterize prior knowledge about the problem (prior) as well as how the accumulation of data updates and refines this knowledge (likelihood). In this minisymposium, we showcase a wide array of Bayesian methods across the various subgroups of mathematical biology. These methods include, but are not limited to: Monte Carlo simulations, data assimilation techniques, Approximate Bayesian Computation, nonlinear mixed effects modeling, joint modeling, individual dynamic predictions, and Bayesian networks. Finally, we provide a forum to discuss the ubiquitous p-value and the corresponding phenomenon of “statistical significance” within the world of Bayesian mathematical biology.
Franz Kuchling
Allen Discovery Center, Tufts University"Uncertainty Minimization as an Adaptive and Evolutionary Imperative in Biology"
Nathanaël Hozé
Université Paris Cité, INSERM, IAME, F-75018, Paris, France"A multi-scale modelling framework to assess the relationship between SARS-CoV-2 viral load and transmission in household studies"
Kathleen Wilkie
Toronto Metropolitan University"Practical Parameter Identifiability and Handling of Censored Data with Bayesian Inference in Models of Tumour Growth"
Ernesto A. B. F. Lima
The University of Texas at Austin"Modeling tumor sensitivity and resistance: a bayesian framework for predicting combination therapies"
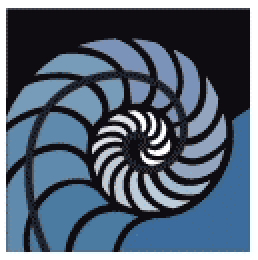