Organizers:
Jennifer Flegg (University of Melbourne), Prof Mat Simpson, Queensland University of Technology
Description:
Modelling whole cells has been identified as a “grand challenge of 21st century science”. However, we currently lack the mathematics, the modelling technologies, and the computational frameworks to understand and predict cellular behaviour. Without mathematical models we cannot understand cellular life, or explore new ways of rationally guiding or designing cellular behaviour. This minisymposium aims to bring together the latest research in methods for whole cell modelling. A variety of methods will be showcased including energy-based modelling methods, machine learning, parameter identifiability, model selection and more. The minisymposium is organised by the Australian Research Council Centre of Excellence in the Mathematical Analysis of Cellular Systems (MACSYS). MACSYS is a 7 year multi-institutional centre that involves several SMB members. The aim of MACSYS is to generate the mathematical, statistical and computational technologies required to make biology predictive; establish mathematical whole cell models for in silico biology as a powerful complement to traditional in vivo and in vitro approaches; tackle fundamental biological problems; and establish a world-leading research and biotechnology translation environment.
Ruth Baker
University of Oxford"Optimal experimental design for parameter estimation in the presence of observation noise"
Yong See Foo
University of Melbourne"Quantifying structural uncertainty in chemical reaction network inference"
Michael Pan
The University of Melbourne"Thermodynamic modelling of membrane transport processes using bond graphs"
Jean (Jiayu) Wen
The Australian National University"Advancing Genomic Foundation Models with Electra-Style Pretraining: Efficient and Interpretable Insights into Gene Regulation"
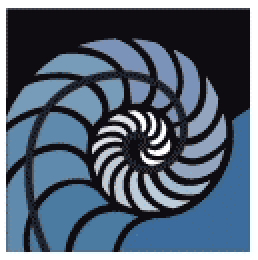