MS04 - MFBM-04
Interaction laws to collective behaviour: Inferring population dynamics
Tuesday, July 15 at 4:00pm
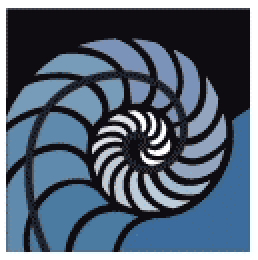
Organizers:
Rebecca Crossley, Stéphanie Abo (University of Oxford), University of Oxford
Description:
This mini-symposium brings together experts from around the globe who are developing cutting-edge techniques for decoding collective behaviour in complex systems, from cellular dynamics to social phenomena. We focus on techniques such as inferring interaction laws from noisy data, designing novel neural network architectures to model emergent behaviour, and applying information-theoretic approaches to understand collective decision-making. The challenge lies not just in quantifying how local interactions aggregate to produce tissue-level or network-level behaviours, but in developing data-driven mathematical frameworks that bridge these scales while preserving essential features. Speakers will present methodological advances in population modelling and statistical learning alongside practical applications in biological systems, ranging from tissue patterning to organised motion. By uniting diverse perspectives, we aim to bridge theory and experiment, foster collaboration, and extract valuable biological insights into how local interaction rules drive coordinated population dynamics.
John Nardini
The College of New Jersey"Decoding agent-based model behavior: novel methods for prediction and global sensitivity analysis"
Jinchao Feng
Great Bay University"A Sparse Bayesian Learning Algorithm for Estimation of Interaction Kernels in Motsch-Tadmor Model"
Seungwoong Ha
Santa Fe Institute"Toward a Data-Centric Understanding of Collective Dynamics"
Ming Guo
Massachusetts Institute of Technology"Collective curvature sensing and fluidity in three-dimensional multicellular systems"
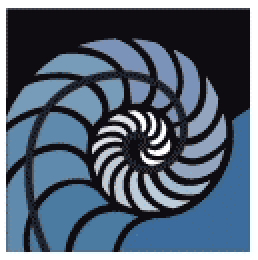