MS05 - MFBM-16
Mathematical Modelling in Disease and Therapy: Integrating Quantitative Frameworks for Deeper Insights
Wednesday, July 16 at 10:20am
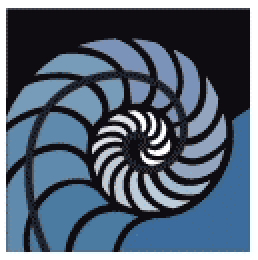
Organizers:
Maria Kleshnina (Queensland University of Technology), Mason Lacy (Queensland University of Technology), Luke Filippini (Queensland University of Technology)
Description:
Mathematical modelling plays a crucial role in advancing our understanding of disease dynamics and optimizing therapeutic strategies. This mini symposium brings together innovative approaches that integrate mathematical frameworks with experimental and clinical data to inform disease modelling and therapy design. The talks will explore how stochastic and continuum models can capture complex cellular behaviours, from immune cell expansion in cancer therapy to anisotropic movement in brain tissue. Algebraic and game-theoretic methods will provide insights into treatment resistance and optimal intervention strategies, while data-driven Boolean network models will shed light on cancer progression at the single-cell level. By combining mathematical techniques with cutting-edge biological data, this symposium will highlight how quantitative approaches can uncover fundamental mechanisms of disease and guide more effective treatment strategies.
Luke Filippini
Queensland University of Technology"Data-informed stochastic frameworks of anisotropic movement in the brain"
Moriah Echlin
Tampere University"Using Single-Cell Data-driven Boolean Network Models to Analyze Prostate Cancer Progression"
Louise Spekking
TU Delft"Improving cancer therapy through migrastatics and estimating tumor composition"
Noa Levi
University of Melbourne"Leveraging algebraic approaches to inform therapeutic intervention"
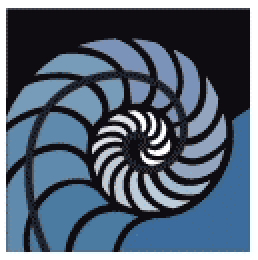