Organizers:
Zhilan Feng (National Science Foundation), John W Glasser, The US Centers for Disease Control and Prevention (CDC)
Description:
Models describe observations, underlying processes, or both. Their utility depends on how well they reproduce observations, those to which they have been fit or others. In descriptive modeling, the parameters of functions with desirable properties are adjusted to minimize discrepancies between predictions and observations. The parameters of mechanistic models cannot be estimated from the observations to which their predictions will be compared. Why? Mechanistic models are hypotheses about the processes giving rise to observations. Fitting their parameters is tantamount to assuming that the underlying processes have been modeled correctly. Hypotheses are tested by comparing their predictions to independent observations. In public health, dynamic models purporting to describe the processes by which pathogens are transmitted among human hosts are simulated under counter-factual conditions to inform policy. Unless the underlying processes have been modeled correctly, their predictions are unreliable. The only way to know if the predictions of mechanistic models are reliable is to compare them to accurate independent observations. The operating characteristics of surveillance systems are rarely considered, if known. Different periods in time-series from such systems are not independent. Averaging the predictions of ad hoc ensembles of such models does not solve the problem. Their undeniable merits notwithstanding, neither do Bayesian methods.
John W Glasser
The US Centers for Disease Control and Prevention (CDC)"Validating a SARS-CoV-2 transmission model"
Wendy S Parker
Virginia Tech"Testing the adequacy-for-purpose of dynamical models"
Michael Y. Li
University of Alberta"Why do models calibrated with data need to be validated?"
Marie Betsy Varughese
Institute of Health Economics"Real-time Validation of Model Projections of Seasonal Influenza in Alberta"
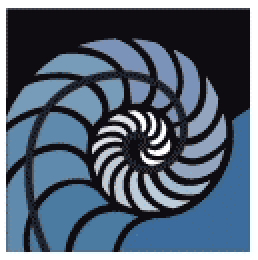