Share this
Organizers:
Kang-Ling Liao (University of Manitoba), Wenrui Hao, Pennsylvania State University
Description:
Mathematical modelling and computation allow for quantitative testing of proposed hypotheses and estimation of important physical and biological parameters. Combining experiments with mathematical modelling allows a rigorous validation of model hypotheses, thereby providing a powerful investigation tool in biology and medicine. The focus of this session will be on applications of mathematics and modelling to the understanding of experiments in biological sciences and medicine.
Weitao Chen
University of California, Riverside"A Mechanochemical Coupled Model to Understand Budding Behavior in Aging Yeast"
Harsh Jain
University of Minnesota Duluth"Looking Beyond Data: Simulating Treatment Outcomes for Unobserved Heterogeneous Populations Using Preclinical Insights"
Leili Shahriyari
University of Massachusetts Amherst"Data Driven QSP Modeling of Cancer: A Step Toward Personalized Treatment"
Nourridine Siewe
Rochester Institute of Technology"Osteoporosis Induced by Cellular Senescence: A Mathematical model"
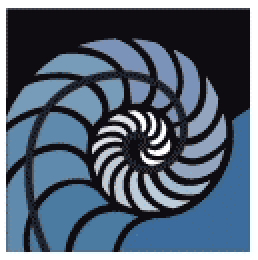