Organizers:
Mohit Kumar Jolly (Indian Institute of Science), Sarthak Sahoo (Indian Institute of Science)
Description:
The ability of cancer cells to dynamically alter their cell-state/phenotype in response to stress, including existing therapies, poses a major impediment to effective cancer treatment. Latest experimental advancements have enabled a better characterization of such cell-state transitions and driven the development of mathematical models that can both offer mechanistic understanding and suggest new potent strategies (combinatorial, sequential, adaptive) for clinical management. This proposed mini-symposium brings together 8 experts across diverse academic backgrounds (oncology, mathematics, engineering), geography (Sweden, India, USA, Canada), career stages (from senior PhD students to full Professors) and genders (4 men, 4 women) to present their latest exciting work in this direction. These experts will discuss how an iterative interdisciplinary crosstalk among multi-scale mathematical models and quantitative experimental and clinical data has unraveled diverse regulatory mechanisms (transcriptional, epigenetic, signalling) contribute to cell-state transitions and consequent heterogeneity in a cell population, and its implications in mediating drug tolerance or resistance for multiple existing therapies, and eventually suggesting new therapeutic strategies that can overcome current clinical challenges.
Rebecca A Bekker
University of Southern California"Modeling Cell-State Dynamics to Unravel and Counteract Immune Suppression in Breast Cancer Immunotherapy"
James Greene
Clarkson University"Understanding therapeutic tolerance through a mathematical model of drug-induced resistance"
Sara Hamis
Uppsala University"Growth rate-driven modelling elucidates phenotypic adaptation in BRAFV600E-mutant melanoma"
Sarthak Sahoo
Indian Institute of Science"Mathematical modelling of multi-axis plasticity in ER+ breast cancer"
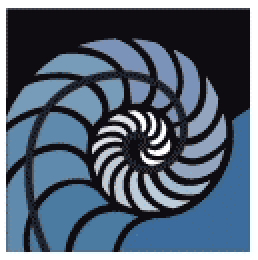