Organizers:
Sungrim Seirin-Lee (Kyoto University/Graduate School of Medicine), Jaekyoung Kim (KAIST), So Miyoshi (Pfizer)
Description:
Another new wave is transforming the landscape of mathematical biology: its full-scale integration into medicine and pharmacy. The time has come for mathematical research, previously focused on theoretical studies, to make significant strides toward practical applications in collaboration with medical doctors and pharmaceutical researchers. This new paradigm aims to directly connect mathematical modeling to real-world treatment, helping patients in the present rather than only laying the groundwork for future possibilities. As this shift takes place, the role of mathematical approaches is becoming increasingly diverse. The complexities of modern medicine and pharmacy demand that mathematical models not only tackle theoretical challenges but also adapt to the nuances of clinical practice and drug development. This requires integrating multiple perspectives, including data-driven methods, predictive modeling, and tools for interpreting biological systems. Mathematics is no longer an auxiliary discipline-it is evolving into a cornerstone of innovation in medical and pharmaceutical research. Through this initiative, we aim to share cutting-edge developments in mathematical medicine and pharmacy, focusing on transformative approaches that link data, models, and real-world implementation. This mini-symposium represents an opportunity to rethink the possibilities of mathematical modeling and to explore its potential for creating tangible solutions in medicine and drug discovery.
Room assignment: Salon 2
Sungrim Seirin-Lee
Kyoto University"Pathological State Inference System based on Skin Eruption Morphology for Personalized Treatments in Dermatology"
Alexander Anderson
Moffitt Cancer Center"Adaptive Therapy from Board to Bench to Bedside and Back Again"
Adrien Hallou
University of Oxford"Spatial mechano-transcriptomics: mapping at single-cell resolution mechanical forces and gene expression in tissues"
Jae Kyoung Kim
KAIST"Improving Biological Predictions: Rethinking Markovian and Diffusion Assumptions"
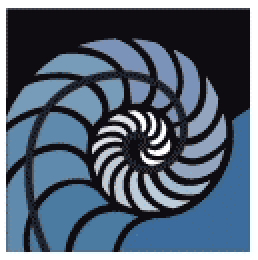