MS03 - MFBM-06
Using Sensitivity Analysis and Uncertainty Quantification to Develop or Improve Biomathematical Models
Tuesday, July 15 at 10:20am
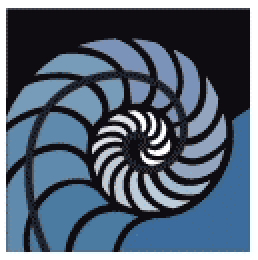
Organizers:
Kelsey Gasior (University of Notre Dame)
Description:
Developing biomathematical models creates a paradox when determining how best to capture a system’s behavior. Too little information creates uninformative models while too many terms results in overparameterization. Further complications occur with the inclusion of measured, experimental data. Understanding the data presented, as well as the model constructed, means that we need to balance the complexity of the model with the information content in the data. In these situations, we often turn to sensitivity analysis. Sensitivity analysis is an important tool when faced with the inability to uniquely determine parameters from existing datasets, known as parameter identifiability. Additionally, it can help us identify the most influential parameters, test the robustness of our models, and improve our understanding of the system’s behavior. The work presented in this minisymposium will discuss the use of novel methodologies in sensitivity analysis and uncertainty quantification to develop biologically relevant biomathematical models. While the biological applications include epithelial intracellular dynamics, bacterial persistence, ecology, and epidemiology, this session aims to connect researchers interested in parameter estimation, sensitivity analysis, and uncertainty quantification. Discussing the development and relevance of these tools on different biological topics will ultimately spark conversations about the needs and future directions for method development.
Samuel Oliver
Swansea University"The role of EMT in Ovarian Cancer: Insights from a Mathematical Model"
Nate Kornetzke
University of New Mexico"Turn down that noise! Uncertainty quantification for stochastic models of emerging infectious pathogens"
Steve Williams
University of California, Merced"Examining models of phenotype selection in populations of bacteria under external predatory stress"
Kelsey Gasior
University of Notre Dame"Comparative Sensitivity Analyses and Modeling the Epithelial Mesenchymal Transition"
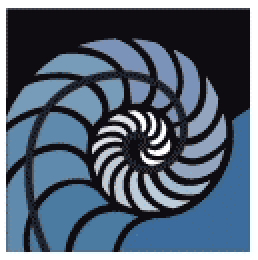