Organizers:
Ioana Bouros (University of Oxford), Alexander Browning, University of Melbourne
Description:
Mathematical models are now indispensable in the interpretation of biological data. Correspondingly, recent years have seen a proliferation of tools for parameter inference and identifiability analysis to aid this interpretation. In this mini-symposium, we aim to showcase diverse methodological approaches to inference and identifiability analysis, in addition to diverse areas of application. In particular, we will highlight problem-specific challenges at the interface of models and data: from epidemiological datasets comprising retrospective observations of a single epidemic, to spatial models where issues of data availability and sparsity are more acute. Through a succession of talks, we will foster discussion and interdisciplinary engagement. We will identify common threads and challenges that underlie the model-data interface, and together identify future areas of research. This mini-symposium aims to facilitate knowledge exchange, inspire novel approaches to integrating models with data, and encourage collaboration between both speakers and the audience. We anticipate a stimulating and fruitful dialogue among mathematical biologists of diverse backgrounds who may not otherwise interact.
Hyukpyo Hong
University of Wisconsin–Madison"Inferring delays in partially observed gene regulation processes"
Hui Jia Farm
University of Oxford"Ensuring parameter identifiability in cardiac cell models is an essential prerequisite for reliable prediction"
Marisa Eisenberg
University of Michigan"Identifiability, uncertainty, and model reduction in mathematical biology"
Tyler Cassidy
University of Leeds"Parameter estimation and identifiability from clinical data in viral dynamics models"
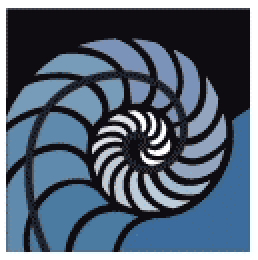