Share this
Organizers:
Tin Phan (Los Alamos National Laboratory), Yun Kang (Arizona State University); Tracy Stepien (University of Florida)
Description:
This session is organized to honor Professor Yang Kuang for his pioneering contributions to mathematical biology, his spirit of collaboration, and his dedication to mentoring 28 PhD students, as well as numerous postdoctoral, master's, and undergraduate researchers. Invited speakers will present cutting-edge research inspired by Dr. Kuang’s foundational work, spanning ecological stoichiometry, delay and partial differential equations, and data-driven modeling in biology. Together, we highlight Dr. Kuang’s achievements and the profound influence his work has on guiding the next generation of researchers in mathematical biology.
Bruce Pell
Lawrence Technological University"Stability Switching Induced by Cross-Immunity in a Two-Strain Virus Competition Model with Wastewater Data Validation"
Erica Rutter
University of California, Merced"Methods for Modeling and Estimating Treatment Heterogeneity in Tumors"
Kyle Nguyen
Sandia National Laboratory"Mathematical modeling of multicellular tumor spheroids quantifies inter-patient and intra-tumor heterogeneity"
Kevin Flores
North Carolina State University"Biologically-informed neural networks for modeling of BKV infection dynamics in renal transplant patients"
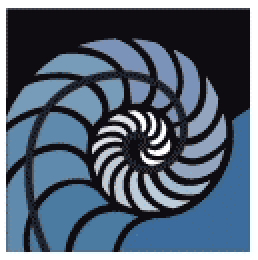