Organizers:
Tomas Helikar (University of Nebraska - Lincoln), Juilee Thakar (Juilee_Thakar@URMC.Rochester.edu) - University of Rochester Medical Center James Glazier (jaglazier@gmail.com) - Indiana University
Description:
Immune Digital Twins (IDTs), virtual models of the human immune system, coupled with periodic real-world data, are a growing focus of precision medicine. Designing control interventions to regulate the immune system requires real-time evaluation of high-dimensional parameter spaces of possible interventions in real-time and the ability to continuously recalibrate models of a changing patient. Because of the sparsity and lack of accuracy of the available experimental data feeds, the lack of first-principles models, the intrinsic stochasticity of the underlying biology, and population heterogeneity building full IDTs presents numerous challenges in model design, parameter identification (in complex agent-based models), uncertainty quantification, forecast deployment and data assimilation for model refinement. Another critical aspect of the development of IDTs is the integration of AI/ML methods with mechanistic models in a variety of roles. Due to the rapidly developing field, we would like to propose and request time for a 5-hour mini-symposium that will focus on modeling and mathematical challenges and achievements related to IDTs. We have secured 10 speakers across disciplines and career stages to cover a wide range of topics, including the use of IDTs in the control of sepsis, respiratory infections, and cancer immunotherapies and approaches to multiscale model construction and parameterization, addressing the aforementioned challenges.
Yi Jiang
Georgia State University"Immunogenic Cell Death: The Key to Unlocking the Potential for Combined Radiation and Immunotherapy"
Josh Loecker
University of Nebraska-Lincoln"Adaptive Analysis of Mechanistic Models using Large Language Models"
Reinhard Laubenbacher
University of Florida"Immune Digital Twins: Foundational Mathematical Challenges"
Gary An
University of Vermont"Curing sepsis with the Critical Illness Digital Twin: An example of the benefit of having a NASEM-compliant Digital Twin"
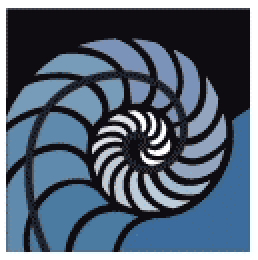