Organizers:
Hye-Won Kang (University of Maryland Baltimore County), Arnab Ganguly, Louisiana State University, aganguly@lsu.edu
Description:
Stochastic modeling has become increasingly popular in the biological sciences, including cell biology, systems biology, and epidemiology. One of the key advantages of stochastic methods is their ability to account for intrinsic fluctuations and uncertainties in experimental outcomes. These methods have proven to be invaluable for analyzing biological systems characterized by random events, stochastic interactions, environmental variability, and low-copy-number molecular species. This minisymposium aims to showcase recent progress in stochastic methods for biochemical reaction networks, spanning both ecological and molecular scales. The sessions will provide a comprehensive overview of the field, covering a broad spectrum of themes that include both applications and methodological advancements. Specific topics of interest include: -New asymptotic results and approximations -Multiscale methods -Statistical inference and learning algorithms for stochastic biological systems -Applications to gene expression, cell signaling, metabolic systems, neuroscience, and epidemiology. A special emphasis will be placed on methods that can be translated into practical tools for broader use in research and applications. By bringing together researchers working on cutting-edge developments, the minisymposium aims to foster interdisciplinary collaboration and inspire innovative approaches and new directions in the study of stochastic processes in biology.
Joshua McGinnis
University of Pennsylvania"Homogenization of a Spatially Extended, Stochastic Ion Channel Model"
Radek Erban
University of Oxford"Chemical Reaction Networks: Systematic Design, Limit Cycles and Spatio-Temporal Modelling"
David Lipshutz
Baylor College of Medicine"Methods for Comparing Sensitivities of Stochastic Neural Networks"
Minji Lee
Ulsan National Institute of Science and Technology"MPUGAT: A Novel Framework for Inferring Dynamic Infectious Disease Transmission with Graph Attention"
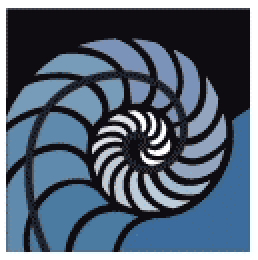