Organizers:
Wenjun Zhao (UBC/Wake Forest University), Khanh Dao Duc (UBC)
Description:
Advances in imaging have for the past few years revolutionized our understanding of biological processes, illustrated by the recent mapping of trillions of human cells, or the explosive rate at which thousands of new protein 3D structures are now determined every year. This unprecedented surge of new biological data yields various mathematical problems and challenges at multiple spatial and time scales for interpreting and analyzing morphological shapes and shape dynamics, which finds close connections to machine learning, statistics, and physics. In this context, our proposed mini-symposium focuses on the mathematical, physical, and statistical aspects of shapes that describe the morphologies of biological structures—from molecules and intracellular organelles to cells, tissues, and organs—and how new modeling tools in this field can help elucidate fundamental biological questions. Intended for a diverse audience of mathematicians, physicists, and computational and experimental biologists, the symposium will feature complementary talks covering multiple aspects of imaging data analysis, ranging from the interplay between cell shapes and fate to new discoveries in protein dynamics through Riemannian geometry. To foster collaboration, share insights, and educate junior scientists, we have invited a diverse panel of participants and speakers from various institutes and career stages, including graduate students, postdocs, and faculty members.
Laurent Younes
JHU"Aligning measures using large deformation diffeomorphic mapping for spatial transcriptomics"
Luis F Pereira
UCSB"Statistical shape analysis with Geomstats"
Qiyu Wang
UBC"Studying SARS-CoV2 spike protein heterogeneity from large Cryo-EM dataset with linear subspace method and path analysis"
Willem Diepeveen
UCLA"Curvature corrected tangent space-based approximation of manifold-valued data and applications in protein dynamics analysis"
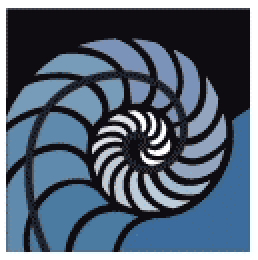