Organizers:
Dhananjay Bhaskar (Yale University), Bernadette Stolz-Pretzer
Description:
The increasing complexity of modern biomedical datasets necessitates advanced mathematical frameworks that reveal intrinsic structures beyond traditional statistical approaches. Geometrical and topological methods provide powerful tools to extract robust and interpretable patterns from high-dimensional, noisy, and multimodal data, enabling more effective data-driven modeling. This minisymposium will explore recent advances at the intersection of geometry, topology, and mathematical modeling, highlighting techniques such as persistent homology, sheaf theory, optimal transport, manifold learning, and geometric deep learning. Speakers will present applications to diverse fields ranging from oncology, liver disease to neuroscience, demonstrating how these methods enhance our understanding of complex biological systems, disease progression, and cellular organization. By fostering interdisciplinary dialogue, this session aims to showcase novel approaches that push the boundaries of data-driven discovery and advance mathematical techniques for biomedical research.
Eunbi Park
Georgia Institute of Technology"Topological data analysis of pattern formation of human induced pluripotent stem cell colonies"
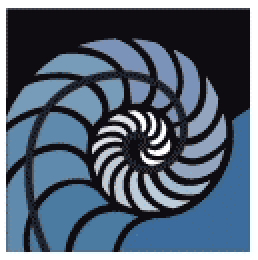