Share this
Brandon Imstepf
University of California, Merced"Accelerating Solutions of Nonlinear PDEs Using Machine Learning: A Case Study with the Network Transport Model"
Youngmin Park
University of Florida"Phase Reduction of Heterogeneous Coupled Oscillators"
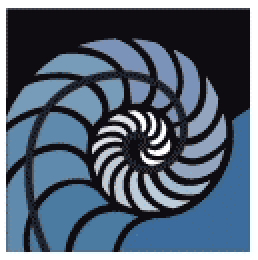