Share this
Gustav Lindwall
Max Planck Institute for Evolutionary Biology"A Mathematical Model for Pseudo-Progression in CAR-T therapy of B-cell Lymphomas"
Rafael Bravo
University of Texas at Austin"Testing the feasibility of estimating the migration to proliferation rate ratio in glioblastoma from single time-point MRI data"
Aaron Li
University of Minnesota"Using a pharmacokinetic ctDNA shedding model to develop a biomarker of tumor response to targeted therapy"
Ruby Nixson
Mathematical Institute, University of Oxford"A structured-PDE approach to targeting a quiescent sub-population under hypoxia and anti-tumour therapies in paediatric glioma."
Reshmi Patel
The University of Texas at Austin"MRI-based mathematical modeling to predict the response of cervical cancer patients to chemoradiation"
Pujan Shrestha
Texas A&M University"An ODE-SDE Model for Ct-DNA dynamics"
Keith Chambers
University of Oxford"Adipocyte-derived lipids promote phenotypic bistability in a structured population model for melanoma growth"
Fabian Spill
University of Birmingham"Regulation of Intra- and Intercellular Metabolite Transport in Cancer Metabolism"
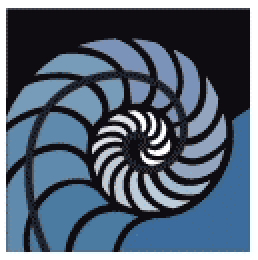