Share this
Ana Forero Pinto
Moffitt Cancer Center/ University of South Florida"An agent-based model with ECM to study the mechanics of DCIS microinvasions"
Chay Paterson
University of Manchester"Wave-like behaviour in cancer evolution"
Nathan Schofield
University of Oxford"Mechanistic modelling of cluster formation in metastatic melanoma"
Sergio Serrano de Haro Ivanez
University of Oxford"Topological quantification of colorectal cancer tissue structure"
Paulameena Shultes
Case Western Reserve University"Cell-Cell Fusion in Cancer: Key In Silico Tumor Evolutionary Behaviors"
Thomas Stiehl
Institute for Computational Biomedicine and Disease Modeling, University Hospital RWTH Aachen, Aachen, Germany & Department of Science and Environment, Roskilde University, Roskilde, Denmark"Computational Modeling of the Aging Human Bone Marrow and Its Role in Blood Cancer Development"
Aisha Turysnkozha
Nazarbayev University"Traveling wave speed and profile of a “go or grow” glioblastoma multiforme model"
Brian Johnson
UC San Diego"Integrating clinical data in mechanistic modeling of colorectal cancer evolution in inflammatory bowel disease"
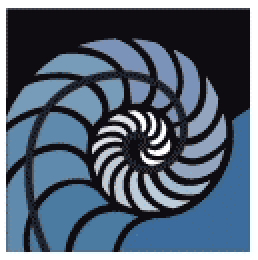