MS04 - ONCO-01
Data-informed mathematical modeling in cancer and development
Tuesday, July 15 at 3:50pm
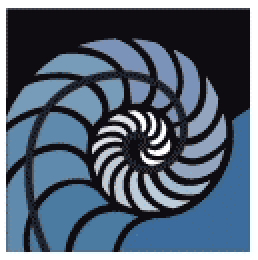
Organizers:
Changhan He (University of California, Irvine), Chengyue Wu, University of Texas MD Anderson Cancer Center
Description:
Understanding and representing the complex dynamics of cancer progression and developmental processes is critical for advancing our knowledge of tumor growth, tissue organization, and treatment response. Data-informed mathematical modeling, integrating frameworks such as differential equations, image-processing techniques, and advanced computational methods, provides powerful tools to capture these intricate biological, chemical, and physical interactions. This mini-symposium aims to unite experts and researchers working on innovative mathematical models and computational approaches, leveraging clinical and experimental data for validation. By bridging mathematical modeling with real-world data, we seek to deepen our understanding of both cancer and developmental biology, fostering collaboration to address key challenges in these interconnected fields.
Lifeng Han
Tulane University"Calibrate a phenotype-structured population model with cell viability data to study drug resistance in cancer treatment"
Wenjun Zhao
Wake Forest University"Dynamical GRN inference via optimal transport"
Qixuan Wang
University of California, Riverside"Hair follicle cell fate regulations and the effect on the follicle growth control"
Axel Almet
University of California, Irvine"Systems modeling of cellular senescence using single-cell transcriptomics"
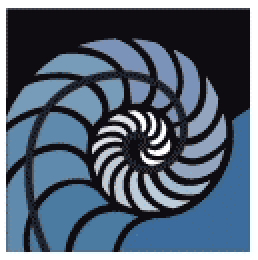