MS03 - ONCO-02
Advances in Optimal Control Methods for Diverse Modeling Frameworks
Tuesday, July 15 at 10:20am in Salon 1
SMB2025 Follow
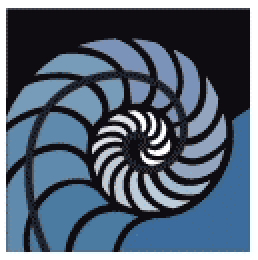
Organizers:
Hannah Anderson (Moffitt Cancer Center), Kasia Rejniak, Moffitt Cancer Center
Description:
Optimal control is an optimization method that has largely been applied to ordinary differential equation models. However, biological systems are often modelled with other modeling frameworks, such as partial differential equations or agent-based models, to examine spatial aspects of the system. This mini-symposium will bring speakers addressing the application of optimal control methods for biological models in the context of each of these diverse modeling frameworks. We will specifically focus on the use of optimal control in treatment optimization, where a range of treatments and diseases will be considered. Optimization aims vary but include addressing drug efficacy, drug toxicity, administrative costs, and spatial constraints of disease growth. We will also evaluate the role of optimal control in the context of digital twins. Thus, this mini-symposium will highlight various modeling frameworks and a broad range of applications in mathematical biology.
Room assignment: Salon 1
Hannah Anderson
Moffitt Cancer Center"Evaluating robustness of an optimized regimen in a virtual murine cohort of bladder cancer"
Christian Parkinson
Michigan State University"Optimal control of a reaction-diffusion epidemic model with noncompliance"
Xinyue Zhao
University of Tennessee Knoxville"Optimal control of free boundary models for tumor growth"
Luis Maria Lopes da Fonseca
University of Florida"Surrogate modeling and control of medical digital twins"
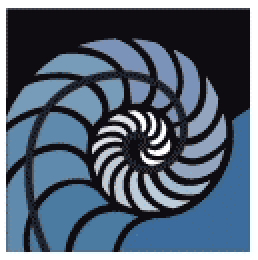