Share this
Organizers:
Jana Gevertz (The College of New Jersey), Thomas Hillen (University of Alberta), Linh Huynh (Dartmouth College)
Description:
Mathematical oncology models describe cancer dynamics using biologically motivated equations that are validated using experimental data. Machine learning models, on the other hand, leverage vast amounts of data to make predictions without necessarily including any a-priori biological knowledge. Mathematical models result in biologically interpretable predictions, whereas machine learning models excel at handling complex, high-dimensional datasets. Thus, work at the interface of modeling and machine learning holds the promise of realizing the advantages of both methods. In this mini-symposium, we will showcase how cancer research benefits from a combined approach of mathematical modeling and machine learning.
Thomas E. Yankeelov
The University of Texas at Austin"Integrating mechanism-based and data driven modeling to predict breast cancer response to neoadjuvant chemotherapy"
Lena Podina
University of Waterloo"Universal Physics-Informed Neural Networks and Their Applications"
Adam L. MacLean
University of Southern California"Dynamic rewiring of cell-cell interaction networks in metastatic TMEs to empower checkpoint inhibition"
Venkata Manem
Centre de Recherche du CHU de Québec; Université Laval, Canada Université Laval, Canada"Beyond the One-Size-Fits-All Paradigm: Leveraging Bioinformatics and AI to Advance Biomarker-Guided Oncology."
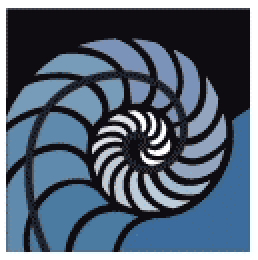