Share this
Organizers:
Jana Gevertz (The College of New Jersey), Thomas Hillen (University of Alberta), Linh Huynh (Dartmouth College)
Description:
Mathematical oncology models describe cancer dynamics using biologically motivated equations that are validated using experimental data. Machine learning models, on the other hand, leverage vast amounts of data to make predictions without necessarily including any a-priori biological knowledge. Mathematical models result in biologically interpretable predictions, whereas machine learning models excel at handling complex, high-dimensional datasets. Thus, work at the interface of modeling and machine learning holds the promise of realizing the advantages of both methods. In this mini-symposium, we will showcase how cancer research benefits from a combined approach of mathematical modeling and machine learning.
John Metzcar
University of Minnesota"Evaluation of mechanistic and machine learning modeling approaches for glioblastoma recurrence prediction using white blood cell dynamics"
Paul Macklin
Indiana University"Integrating high-throughput exploration and learning with agent-based models of cancer"
Kit Gallagher
University of Oxford, Moffitt Cancer Center"Predicting Treatment Outcomes from Adaptive Therapy — A New Mathematical Biomarker"
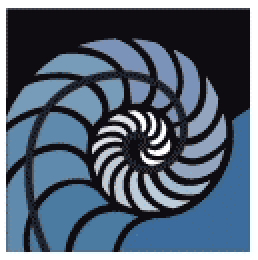