Organizers:
Einar Bjarki Gunnarsson (Science Institute, University of Iceland), Maximilian Strobl (Cleveland Clinic, USA, stroblm@ccf.org)
Description:
Drug resistance is a common reason for treatment failure in cancer. While we have learned much about the molecular mechanisms of resistance, less is known about the evolutionary processes by which resistant populations arise and expand, and how anti-cancer drugs influence these processes. Yet, such knowledge is crucial for informing when and how to treat to delay or avert resistance. In this mini-symposium, we are interested in the role of non-genetic mechanisms in resistance evolution, based on mounting evidence that anti-cancer drugs designed to kill cancer cells can simultaneously induce the adoption of non-genetic cell states capable of drug persistence or immune evasion. This evidence challenges the traditional Darwinian view of resistance evolution and confounds conventional high-dose therapy regimens. At the same time, the reversible nature of non-genetic mechanisms creates opportunities for delaying resistance through intermittent or adaptive therapy. We will hear talks from an interdisciplinary panel of experimental, data-driven and theoretical researchers intended to stimulate a conversation around the design of experiments for interrogating drug-induced persistence, the development of mathematical models and associated parameter inference methods, and mathematical model-informed optimal drug scheduling to tackle resistance. The talks will address both targeted therapies and immunotherapies, covering two major types of modern anti-cancer treatment.
Einar Bjarki Gunnarsson
Science Institute, University of Iceland"Decoding drug-induced persistence: Integrating theory with experiments and optimizing dosing protocols"
Mattia Corigliano
IFOM - The AIRC Institute of Molecular Oncology, Milan, Italy"Optimal treatment for drug-induced cancer persisters involves release periods and intermediate drug doses"
Irina Kareva
Northeastern University"Dosing Strategies for Bispecifics with a Bell-Shaped Efficacy Curve: What Looks Like Resistance May Be Corrected Through Schedule Adjustments"
Tatiana Miti
Moffitt Cancer Center & Research Institute"ABM studies on the role of the drug-sheltering effects of stroma on the emergence of resistance"
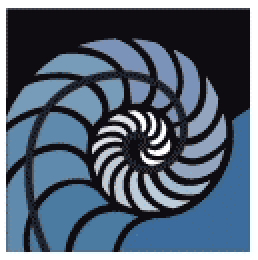