Organizers:
Einar Bjarki Gunnarsson (Science Institute, University of Iceland), Maximilian Strobl (Cleveland Clinic, USA, stroblm@ccf.org)
Description:
Drug resistance is a common reason for treatment failure in cancer. While we have learned much about the molecular mechanisms of resistance, less is known about the evolutionary processes by which resistant populations arise and expand, and how anti-cancer drugs influence these processes. Yet, such knowledge is crucial for informing when and how to treat to delay or avert resistance. In this mini-symposium, we are interested in the role of non-genetic mechanisms in resistance evolution, based on mounting evidence that anti-cancer drugs designed to kill cancer cells can simultaneously induce the adoption of non-genetic cell states capable of drug persistence or immune evasion. This evidence challenges the traditional Darwinian view of resistance evolution and confounds conventional high-dose therapy regimens. At the same time, the reversible nature of non-genetic mechanisms creates opportunities for delaying resistance through intermittent or adaptive therapy. We will hear talks from an interdisciplinary panel of experimental, data-driven and theoretical researchers intended to stimulate a conversation around the design of experiments for interrogating drug-induced persistence, the development of mathematical models and associated parameter inference methods, and mathematical model-informed optimal drug scheduling to tackle resistance. The talks will address both targeted therapies and immunotherapies, covering two major types of modern anti-cancer treatment.
Room assignment: Salon 13/14
Jana Gevertz
The College of New Jersey"Mitigating non-genetic resistance to checkpoint inhibition based on multiple states of exhaustion"
Raymond Ng
University of Pennsylvania"The role of tumor gene expression variability in evading CD8+ T cells"
Chenyu Wu
University of Minnesota"A statistical framework for detecting therapy-induced resistance from drug screens"
Michael Cotner
The University of Texas at Austin"Tracking Resistance to Targeted Therapy in TNBC with Cell Barcodes"
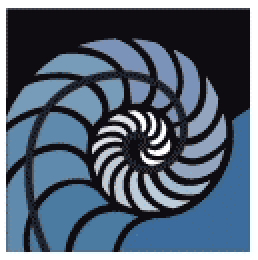