Organizers:
Ashlee N. Ford Versypt (University at Buffalo, State University of New York), John Metzcar, University of Minnesota
Description:
Cancer systems biology aims to understand cancer as an integrated system of genes, proteins, cells, tissue microenvironments, treatments, whole body physiology, and environmental factors. Cancer is connected in interacting networks across scales, rather than an entity of isolated molecular and cellular components. Many mathematical approaches are powerful tools for both mechanistic modeling and data-driven analyses that enable new insights into various scales of cancer systems biology. A growing community of interdisciplinary researchers in cancer systems biology have assembled at a meeting called Systems Approaches to Cancer Biology, held five times since 2016. A motivation for this minisymposium is to feature researchers from the cancer systems biology community to broaden the exposure and connections with the mathematical oncology and wider community through SMB. This minisymposium will focus on the mathematical methods and models applied to understand the complexities of cancer systems biology. Potential topics include: - Dynamics for cancer cell signaling and metabolic interactions - Tensor-based/multilinear modeling for integrating cancer omics data - Agent-based models of interactions in the tumor microenvironments - Boolean network modeling for cancer cell functions - Machine learning to infer targetable pathways and treatment options
Ashlee N. Ford Versypt
University at Buffalo, State University of New York"Agent-Based Modeling of the Transwell Migration Assay to Inform Tumor-Immune Microenvironment Simulations"
Aaron Meyer
University of California, Los Angeles"Bridging single cell features to the tissue and patient scale with tensor modeling"
Erzsébet Ravasz Regan
The College of Wooster"Cell Interrupted — Modular Boolean Modeling of the Coordination between Mitochondrial Dysfunction-Associated Senescence, Cell Cycle Control and the Epithelial to Mesenchymal Transition"
Stacey D. Finley
University of Southern California"Systems biology modeling and analyses of metabolic phenotypes in the tumor microenvironment"
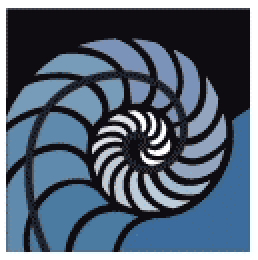