Share this
Organizers:
Kang-Ling Liao (University of Manitoba), Wenrui Hao, Pennsylvania State University
Description:
Mathematical modelling and computation allow for quantitative testing of proposed hypotheses and estimation of important physical and biological parameters. Combining experiments with mathematical modelling allows a rigorous validation of model hypotheses, thereby providing a powerful investigation tool in biology and medicine. The focus of this session will be on applications of mathematics and modelling to the understanding of experiments in biological sciences and medicine.
Room assignment: Salon 5
Kang-Ling Liao
University of Manitoba"Mathematical Modeling of Breast Cancer Treatment with Radiation, Anti-estrogen, and Immune Checkpoint Inhibitor"
Tracy Stepien
University of Florida"Modeling Tumor-Immune Interactions in the Glioblastoma Microenvironment"
Harsh Jain
University of Minnesota Duluth"Looking Beyond Data: Simulating Treatment Outcomes for Unobserved Heterogeneous Populations Using Preclinical Insights"
Negar Mohammadnejad
University of Alberta"Strategies for Optimizing the Efficacy of Oncolytic Virus–Immune System Interactions"
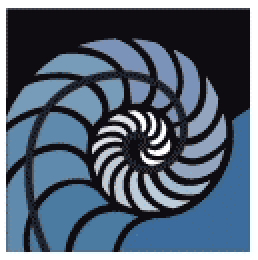