Organizers:
Hye-Won Kang (University of Maryland Baltimore County), Arnab Ganguly, Louisiana State University, aganguly@lsu.edu
Description:
Stochastic modeling has become increasingly popular in the biological sciences, including cell biology, systems biology, and epidemiology. One of the key advantages of stochastic methods is their ability to account for intrinsic fluctuations and uncertainties in experimental outcomes. These methods have proven to be invaluable for analyzing biological systems characterized by random events, stochastic interactions, environmental variability, and low-copy-number molecular species. This minisymposium aims to showcase recent progress in stochastic methods for biochemical reaction networks, spanning both ecological and molecular scales. The sessions will provide a comprehensive overview of the field, covering a broad spectrum of themes that include both applications and methodological advancements. Specific topics of interest include: -New asymptotic results and approximations -Multiscale methods -Statistical inference and learning algorithms for stochastic biological systems -Applications to gene expression, cell signaling, metabolic systems, neuroscience, and epidemiology. A special emphasis will be placed on methods that can be translated into practical tools for broader use in research and applications. By bringing together researchers working on cutting-edge developments, the minisymposium aims to foster interdisciplinary collaboration and inspire innovative approaches and new directions in the study of stochastic processes in biology.
Suzanne Sindi
University of California Merced"Scalable Bayesian Discovery of Chemical Reaction Networks from Fully Observed Stochastic Dynamics"
Muruhan Rathinam
University of Maryland Baltimore County"Stochastic Filtering of Reaction Networks"
Arnab Ganguly
Louisiana State University"Multiscale Enzyme Kinetic Reactions: Stochastic Averaging and Statistical Inference"
Boseung Choi
Korea University Sejong Campus"Statistical Inference Method for Identifying the Stochastic Chemical Kinetics Using Logistic Regression"
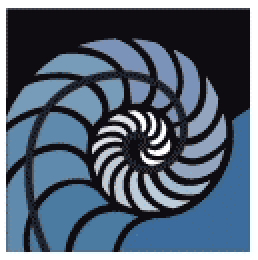