Organizers:
Guillermo Lorenzo (University of A Coruna (Spain)), Chengyue Wu (The University of Texas MD Anderson Cancer Center, US), Ernesto A. B. F. Lima (The University of Texas at Austin, US)
Description:
The overall goal of this minisymposium is to present and discuss recent developments of digital twin technologies to (i) address the personalization of clinical management of cancers, and (ii) advance the research on biophysical mechanisms underlying these pathologies from the micro to the macroscale. A digital twin can be defined as a virtual representation of a physical object by means of a computational model that continuously assimilates object-specific data to enable-decision making about the physical object based on its current and future states. Digital twins have a widespread development in multiple areas of engineering and they have also been proven useful in several areas of medicine, such as surgical planning, cardiovascular disease interventions, and glucose monitoring in diabetic patients. Given the increasing success of computational models to predict the development of cancer and its response to treatments, these models could be employed to construct digital twins to support the optimal diagnosis, monitoring, and treatment of individual patients as well as to assist the research of this disease in vitro, in vivo, and in silico. The talks in this minisymposium will present recent efforts in building digital twins for the clinical management of cancers and the (pre)clinical research of these diseases, as well as ongoing work on practical mathematical models and computational methods for the development of these predictive technologies.
Thomas E. Yankeelov
The University of Texas at Austin"A practical computational framework for systematically investigating alternative treatment strategies for cancer"
Maximilian Strobl
Cleveland Clinic"What pre-clinical experiments can teach us about digital twins for personalized cancer treatment scheduling"
Renee Brady-Nicholls
H. Lee Moffitt Cancer Center & Research Institute"Investigating Response Differences between African American and European American Prostate Cancer Patients Through an In Silico Study"
Fatemeh Beigmohammadi
Université de Montréal"Efficient methods for generating virtual patient cohorts using trajectory-matching ABC-MCMC"
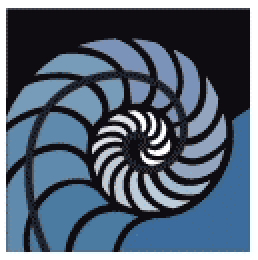