Organizers:
Guillermo Lorenzo (University of A Coruna (Spain)), Chengyue Wu (The University of Texas MD Anderson Cancer Center, US), Ernesto A. B. F. Lima (The University of Texas at Austin, US)
Description:
The overall goal of this minisymposium is to present and discuss recent developments of digital twin technologies to (i) address the personalization of clinical management of cancers, and (ii) advance the research on biophysical mechanisms underlying these pathologies from the micro to the macroscale. A digital twin can be defined as a virtual representation of a physical object by means of a computational model that continuously assimilates object-specific data to enable-decision making about the physical object based on its current and future states. Digital twins have a widespread development in multiple areas of engineering and they have also been proven useful in several areas of medicine, such as surgical planning, cardiovascular disease interventions, and glucose monitoring in diabetic patients. Given the increasing success of computational models to predict the development of cancer and its response to treatments, these models could be employed to construct digital twins to support the optimal diagnosis, monitoring, and treatment of individual patients as well as to assist the research of this disease in vitro, in vivo, and in silico. The talks in this minisymposium will present recent efforts in building digital twins for the clinical management of cancers and the (pre)clinical research of these diseases, as well as ongoing work on practical mathematical models and computational methods for the development of these predictive technologies.
Heber L. Rocha
Indiana University"Agent-Based Modeling of Cancer Drug Response with PKPD Calibration Challenges and Personalized Modeling"
Marianna Cerasuolo
University of Sussex"Mathematical and Statistical Insights into Gut Microbiota–Phytocannabinoid–Diet Interplay in Prostate Cancer Progression in Mice"
Guillermo Lorenzo
University of A Coruna"Patient-specific forecasting of prostate cancer progression to higher-risk disease during active surveillance"
David A. Hormuth, II
The University of Texas at Austin Texas"Image-based habitat dynamics in patients with head and neck cancer undergoing radiotherapy"
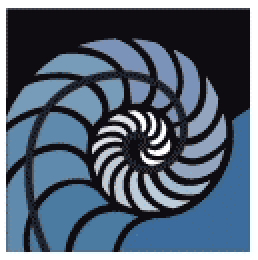