Organizers:
Veronica Ciocanel (Duke University), Hye-Won Kang, University of Maryland Baltimore County
Description:
This minisymposium aims to highlight recent advances in emerging areas and novel mathematical approaches for addressing biological questions. The sessions will cover a broad spectrum of themes that integrate data-driven mathematical modeling and real-world applications. Topics covered include advances in stochastic and continuum modeling of molecular and regulatory mechanisms in cells, network dynamics in infectious disease and ecological systems, and data-driven methods for sleep homeostasis and disorders. These sessions will also celebrate the impactful mathematical biology research of former Mathematical Biosciences Institute (MBI) postdocs and visitors whose career was influenced by their time and connections made while at the MBI.
Scott McKinley
Tulane University"Robust inference and model selection for particle tracking in live cells"
Peter Kramer
Rensselaer Polytechnic Institute"Molecular Mechanisms in Actively Driven Passively Crosslinked Microtubule Pairs"
Yangyang Wang
Brandeis University"A conceptual framework for modeling a latching mechanism for cell cycle regulation"
Kasia Rejniak
Moffitt Cancer Center"Data-driven models for guiding adoptive cell therapies in bladder cancer"
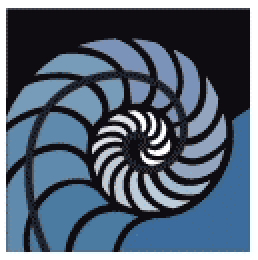