Organizers:
Veronica Ciocanel (Duke University), Hye-Won Kang, University of Maryland Baltimore County
Description:
This minisymposium aims to highlight recent advances in emerging areas and novel mathematical approaches for addressing biological questions. The sessions will cover a broad spectrum of themes that integrate data-driven mathematical modeling and real-world applications. Topics covered include advances in stochastic and continuum modeling of molecular and regulatory mechanisms in cells, network dynamics in infectious disease and ecological systems, and data-driven methods for sleep homeostasis and disorders. These sessions will also celebrate the impactful mathematical biology research of former Mathematical Biosciences Institute (MBI) postdocs and visitors whose career was influenced by their time and connections made while at the MBI.
Grzegorz Rempala
The Ohio State University"Modeling Epidemics on Networks"
Paul Hurtado
University of Nevada, Reno"SIER-type ODE models with phase-type latent and infectious period distributions"
Deena Schmidt
University of Nevada, Reno"Modeling network formation in ecological systems"
Katarzyna Rejniak
Moffitt Cancer Center"Data-driven models for guiding adoptive cell therapies in bladder cancer"
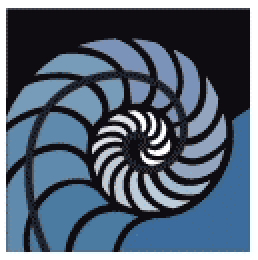